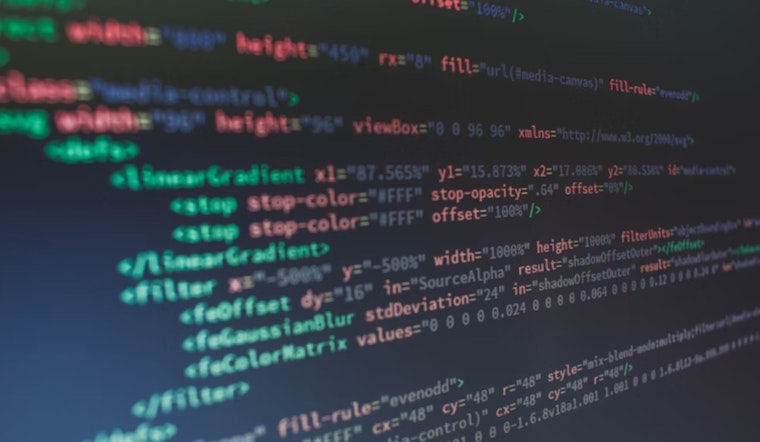
Researchers at MIT, alongside international collaborators, have developed a novel technique that enhances the reasoning prowess of those behind-the-scenes workhorses known as large language models, which include the likes of ChatGPT. This new approach, as detailed on MIT's official news portal, uses what’s known as natural language embedded programs, cleverly merging the complexities of human language with the precision of programming, offering a new level of transparency and trust in how AI solves problems.
Straddling the realms of language and computation, large language models play a pivotal role in applications ranging from drafting complex documents to crunching through data analysis, though they've historically stumbled when it comes to tasks demanding a blend of verbal agility and numerical savvy—imagine an adept translator that trips over basic arithmetic. While current Large Language Models like GPT-4 can churn out whole programs, they oftentimes nest these within strands of natural language, which means they can make errors in both the logic and the conclusions of their code-laden rambles but researchers from MIT are taking distinct strides to leapfrog over these hurdles— we're looking at AI that could, for example, not only remember when U.S. presidents were born but also nail down which ones celebrated their birthdays on a hump day, if they were elected after the mid-20th century.
MIT postdoc Hongyin Luo, a co-lead author of the paper on natural language embedded programs, encapsulated the essence of the innovation by saying, "We want AI to perform complex reasoning in a way that is transparent and trustworthy. There is still a long way to go, but we have shown that combining the capabilities of programming and natural language in large language models is a very good potential first step toward a future where people can fully understand and trust what is going on inside their AI model." Alongside Luo are Tianhua Zhang, Jiaxin Ge, Yoon Kim, James Glass, and other contributors to this trailblazing study, slated for presentation at the forthcoming Annual Conference of the North American Chapter of the Association for Computational Linguistics.
The method developed involves coaxing a language model to piece together a Python program in response to a user's query which could be as simple as calculating the nth prime number or as tricky as tracking objects through a series of shuffles; the program then delivers results dressed in everyday language so users don't need to parse code to get the information they seek. It's akin to having a bridge between digital fluency and human interaction, which has already showcased its mettle by surpassing the 90% threshold in accuracy on various symbolic reasoning tasks that's not just a stairstep but a full staircase above traditional prompt-based approaches that would have language models twiddling their digital thumbs, perplexed.
The implications of this advancement are multi-layered, potentially enhancing smaller models without the need for costly retraining, and adding a privacy shield since these natural language embedded programs programs run locally—no need to send sensitive info into the cloud universe. As Hongyin Luo frankly puts it, "There is no magic here. We do not have a more expensive or fancy language model. All we do is use program generation instead of natural language lasagna generation, and we can make it perform significantly better." While the technique currently flexes its best moves with larger models, the team at MIT is looking into ways to make this technology play nice with its smaller counterparts, as well, setting the stage for a more scalable and effective future in AI reasoning.